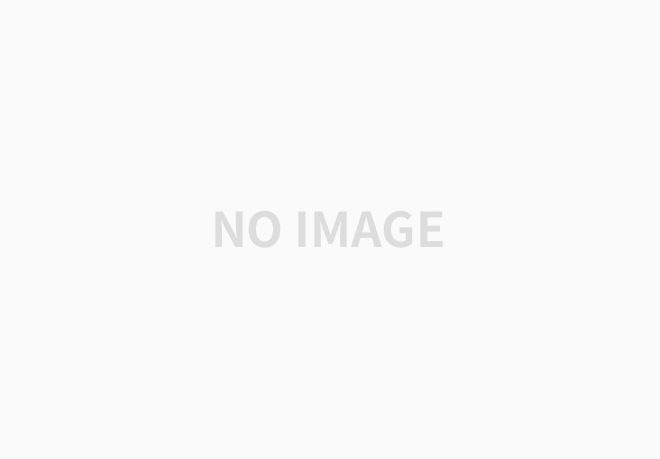
데이터셋의 비교는 기본적으로 두 확률변수의 linear관계를 확인하는 covariance 를 보는 것이다. 하지만 covariance 를 비교하면 데이터의 scale에 따라 다른 결과를 주게된다. 이를 분산으로 나누어 normailzed 값이 correlation coefficient이며, 데이터의 방향과 정도를 확인할 수 있다.
Correlation coefficient를 구하는 3가지 방법에 대해 정리해 본다.
- Pearson's correlation coefficient ( value )
- Spearman's correlation coefficient ( rank )
- Kendall rank correlation coefficient ( rank )
Pearson's correlation coefficient
* 가정 : 1) 두 비교 데이터의 독립성 2) 선형관계 3) 동분산성 (Homoscedasticity)
- 주로 두 데이터의 선형관계를 확인하는데 많이 쓰인다. ( -1 ≤ r ≤ 1 )
Spearman's correlation coefficient
* 가정 : 1) ordinality 2) monotonically increased
- Ranking 으로 나타낼 수 있는 데이터에 사용하고, rank가 어떻게 변했는지에 대해 비교하는 방법이다.
Kendall rank correlation coefficient
* 가정 : 1) ordinality
- 데이터를 내림차순으로 정렬했을 때, 비교하고자 하는 데이터의 순위가 어떤지 비교하는 방법이다.
- 비교 가능한 전체 쌍은 n개에서 2개를 선택하는 조합이기 때문에 nC2 로 나누어 준다.
Spearman 과 Kendall 은 generalized correlation coefficient 의 특별한 경우이다.
https://en.wikipedia.org/wiki/Rank_correlation#General_correlation_coefficient
Reference
- https://en.wikipedia.org/wiki/Pearson_correlation_coefficient
- https://en.wikipedia.org/wiki/Rank_correlation
- https://www.statisticshowto.com/kendalls-tau/
- https://en.wikipedia.org/wiki/Homoscedasticity
- https://en.wikipedia.org/wiki/Variance
- https://en.wikipedia.org/wiki/Random_variable
- https://drhongdatanote.tistory.com/49
'Statistics' 카테고리의 다른 글
Mean vs. Variance (0) | 2021.10.20 |
---|---|
Count data distribution (0) | 2021.10.17 |
Regression error (0) | 2021.07.04 |
Multiple test correction (0) | 2021.06.10 |
ANOVA (0) | 2021.06.08 |
댓글