Population genetics 글에 이어 Coalescent theory에 대해서 자세히 알아보자.
Wikipedia에 따르면, Coalescent theory란 모집단에서 표본으로 추출한 유전자 변형이 어떻게 공통의 조상으로부터 비롯되었을 수 있는지를 보여주는 model이다. 가장 간단한 경우, coalescent theory는 no recombination, no natural selection, no gene flow, no population structure를 가정하며, 이는 각 변종이 한 세대에서 다음 세대로 똑같이 전해졌을 가능성이 크다는 것을 의미한다. 알아야 할 점은, coalescent theory는 tree reconstruction method가 아니라 sampling method이다. (how to build the coalescent = how to model the relationships of individuals within a sample.)
Coalescent 확률은 현재 세대에서 이전 세대로 거슬러 올라갈 때 coalescence가 일어날 확률이다.
한 세대에 N명이 있을 때, 총 2N의 유전자(allele)를 가지게 된다. 2명이 같은 부모중 한명으로부터 유전자를 받을 확률은 이전 세대의 2N개의 유전자가 있고, 그 중에 하나가 선택되는 것이기 때문에 P=1/(2N)이 된다. 그리고 같은 부모로부터 유전자를 받지 못 할 확률은 Q=1-P=1-1/(2N)이 된다. 즉, coalescence-event가 일어나지 않을 확률이 Q라는 이야기이다.
2-Coalescent, 즉 2-lineage일 경우의 확률은 coalescence가 일어난 시간이 t였다면, 처음 coalescence가 일어난 확률과 t-1세대까지 coalescence가 일어나지 않는 확률의 곱으로 나타난다.
이러한 확률을 가질 때, coalescence가 일어나는 평균 세대(the expectation for the time of the MRCA)는 coalescence가 일어나는 확률의 역수로 구할 수 있다. 예를 들어 한 세대에 10명이 있다면, 평균 20세대를 올라가야 MRCA를 찾을 수 있다는 말이다.
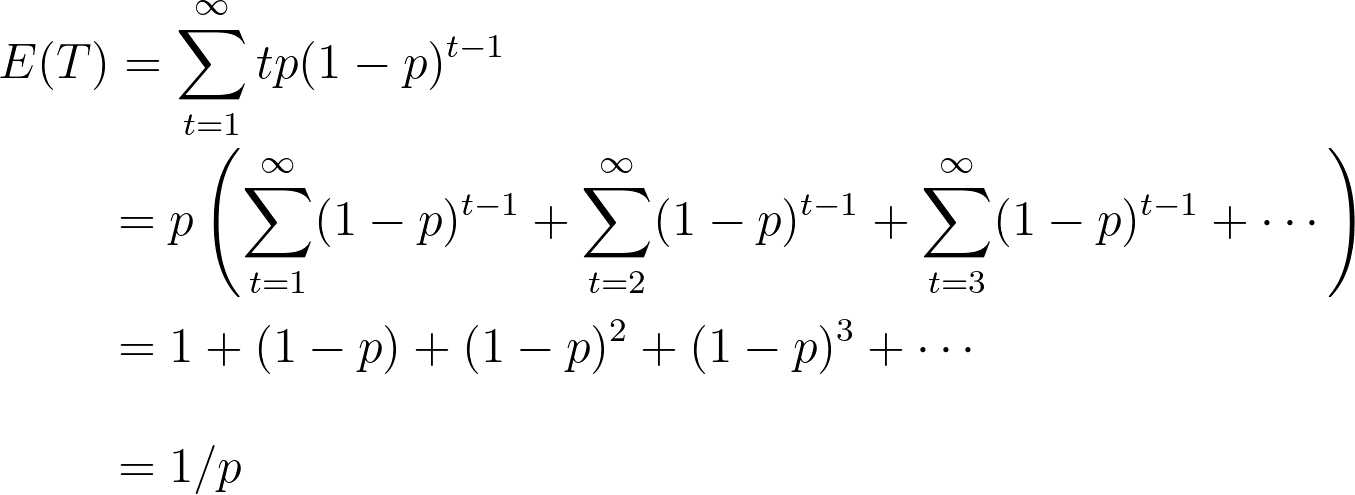

where
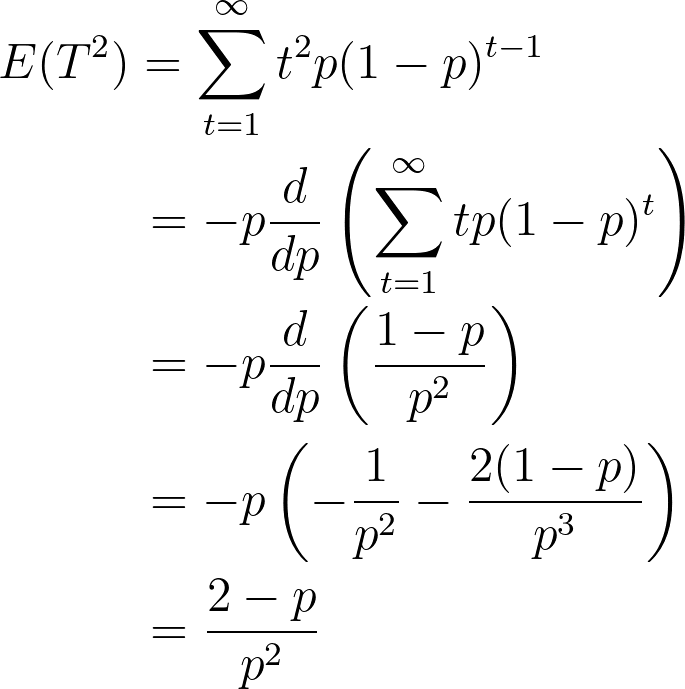
그렇다면 k명의 sample이 있을 때, coalescence가 일어날 확률은 어떻게 될까? Coalescence가 일어날 확률을 구하는 것보다 일어나지 않을 확률을 먼저 구하는 것이 더 쉽다.
coalescence가 일어나지 않을 확률을 구해보자. 다시 말해, 같은 부모를 가지지 않을 확률을 구해 보자. 3-lineage가 있을 때, 첫 번째 sample의 부모 유전자가 유전될(matchinge될) 확률은 1이다. 두 번째 sample은 2N개의 유전자에서 첫 번째 선택된 부모의 유전자를 제외하면 2N-1이 되어 확률은 (2N-1)/2N이 되고, 세 번째 sample은 또 다른 부모의 유전자를 받는다고 하면 앞서의 2유전자를 제외하고 (2N-2)/2N이 된다. 동시에 일어나기 때문에 곱해주면 같은 부모를 가지지 않을 확률, 즉 coalescent가 일어나지 않을 확률이 된다.
이것을 확장하여 k-lineage가 있을 경우, coalescence가 일어나지 않을 확률은 다음과 같고, N이 충분히 크면 근사할 수 있다.
t세대까지 coalescence가 일어나지 않으면 t번의 곱으로 나타낼 수 있고, exponential로 근사된다.
Coalescence가 일어날 확률은 p(coalescence)=1-p(no coalescence) 로 구할 수 있다.
Average coalescent time in units of 2N generations, 즉 2N 세대에서의 coalescence 기대값은 다음과 같다.
k-lineage의 coalescence가 끝나기까지의 시간은 각 coalescent time의 합으로 구한다.
Mutation이 일어나는 Segregating site, S,에 대한 전체 기대값은 다음과 같으며, mutation rate를 식의 계수로 정의한다. µ는 한 세대에 한 사이트에서 mutation이 일어날 확률이다.
θ로 정의한 mutation rate는 recombination rate로도 정의된다.
Effective_population_size : Best appoximates the real population (or the model)
Markov Chain Monte Carlo
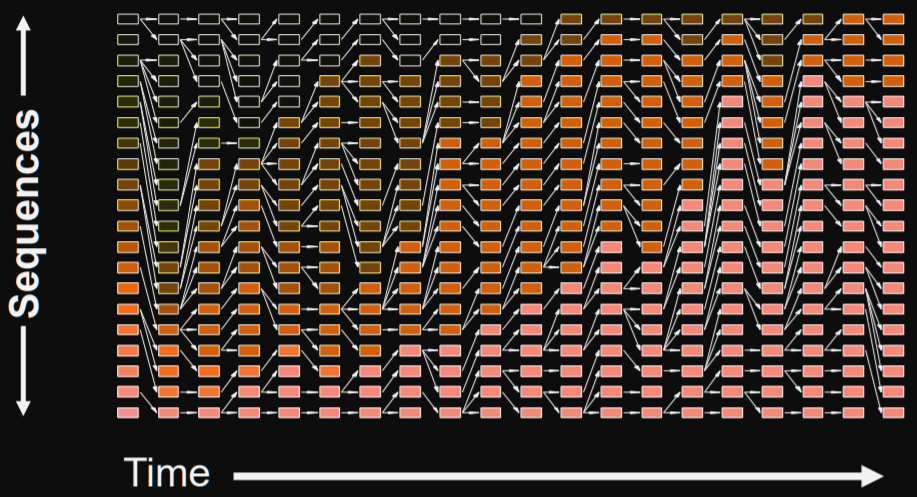
Reference
- http://www.incodom.kr/Population_genetics
- http://genetics.wustl.edu/bio5488/files/2016/03/Bio5488_PopGen_2016_Lecture1.pdf
- http://genetics.wustl.edu/bio5488/files/2016/03/Bio5488_PopGen_2016_Lecture2.pdf
- http://www.sfu.ca/biology/courses/bisc869/869_lectures/MHP_Coalescent.pdf
- http://ib.berkeley.edu/courses/ib200/2016/labs/11/coalescent_lecture.pdf
- https://cme.h-its.org/exelixis/web/teaching/seminarSlides/example2.pdf
- https://en.wikipedia.org/wiki/Coalescent_theory
- https://genome.sph.umich.edu/w/images/4/4f/666.04_-_The_Coalescent.pdf
- https://cme.h-its.org/exelixis/web/teaching/lectures/lecture14.pdf
'Study' 카테고리의 다른 글
Genetic map (1) | 2020.07.31 |
---|---|
Markov chain (0) | 2020.07.24 |
Population genetics (0) | 2020.06.25 |
Phasing (0) | 2020.06.17 |
Random Walk (0) | 2020.06.17 |
댓글